A new feature: Engagement scoring
At GetAccept, our goal is to help our customers make smarter decisions to close more deals faster. We have been working with machine learning for some time now so that we can provide more tangible results for our customers.
With this, we are excited to introduce our newest feature, Engagement Scoring, a pivotal tool to help our customers understand the likelihood of closing a deal and provide insights into the factors resulting in a particular score.
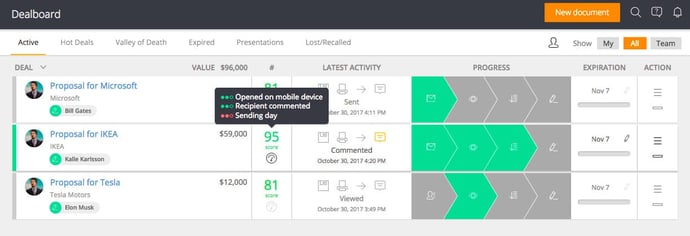
Why
Data is crucial when designing successful and personalized engagement with our customers. A data driven approach is key to taking important decisions and developing sales strategies. By combining the abundant data we have with machine learning, we are helping to develop effective sales strategies in ways humans alone cannot.
Furthermore, predictability acts as the bedrock of any sales organization. It is critical to know the health of each deal and understand which ones have a higher likelihood of closing than others. This information is imperative when forecasting and helping sales professionals decide which deals to channel their energy and efforts toward.
What if you could tailor how you approach the deal and prime it for the win without relying on intuition alone? What if this prediction could learn and improve over time as you close more deals? At GetAccept, we collect data connected to every sent sales document, which is why we are looking into the field of AI and machine learning to help our customers become better sales professionals.
What
The GetAccept Engagement Scoring uses predictive modeling and machine learning to provide insights on where the deal stands at a certain point in time and the likelihood of it being signed. The higher the score, the higher the likelihood of the deal closing. It also provides guidance on targeted actions sales professionals can take to close deals more efficiently. It acts as a sales coach in the most important phase of the deal; the closing phase.
How
From a technical perspective, our Engagement Scoring uses a classification algorithm, namely logistic regression, to train the model and predict whether a deal will be signed (=1) or not (=0), that is, y ∈ {0, 1}.
.jpg)
Historical data, consisting of thousands of deals with multiple parameters, where the outcomes were already known, was used to train the model. The data was split into two sets; training and testing. The model was trained using the training set with 89% accuracy and a precision of 87%. Predictions were then calculated on the test set.
Precision was especially important for our application to accurately predict all the deals that will be signed and eliminate any occurrences of false positives. The model is trained on a daily basis as more and more data gets continuously added into the historical dataset.
The scores and the reasons behind the scoring is predicted for any new deal that is fed into the model. For example, one specific document that we tested had a high score that was strongly correlated to a shorter view time and a fewer number of reminders sent.
Another document we analyzed which received a much lower score, strongly correlated to a longer view time and the fact the sender delivered the document towards the latter half of the week (chances of the recipient opening the document on Friday are lower than on Monday or Tuesday). These reasons are unique to each individual account and will evolve over time as the model is fed more and more data.
Conclusion
A lot of factors go into understanding what attributes to closing deals, and the exciting thing is, we have only scratched the surface with the first version of Engagement Scoring.
Talking about how machine learning is connected to the software is easy, but to incorporate it into the product demands a much more holistic approach, one which involves creating the data pipeline, extensive exploratory data analysis, developing the model, and planning the roadmap.
It's easy to end up with a bunch of "if-then statements" and depend on your gut feeling rather than relying on a data-driven model. However, through our Engagement Scoring, we hope to challenge this instinct and demonstrate the value of a data-driven closing process.
With this new feature, sales professionals can easily see which documents are more likely to be signed, and which may need some extra attention. It not only gives you a score to set order to your deals, but also the reasons to why they're receiving the scores.
This knowledge acts as a sales coach when beginning to build best practices for delivering and closing your sales collateral. In the near future, it will suggest, and even automate, the next best steps to help you close more deals.
The more you use engagement scoring, the smarter it gets. The smarter it gets, the more deals you'll close!